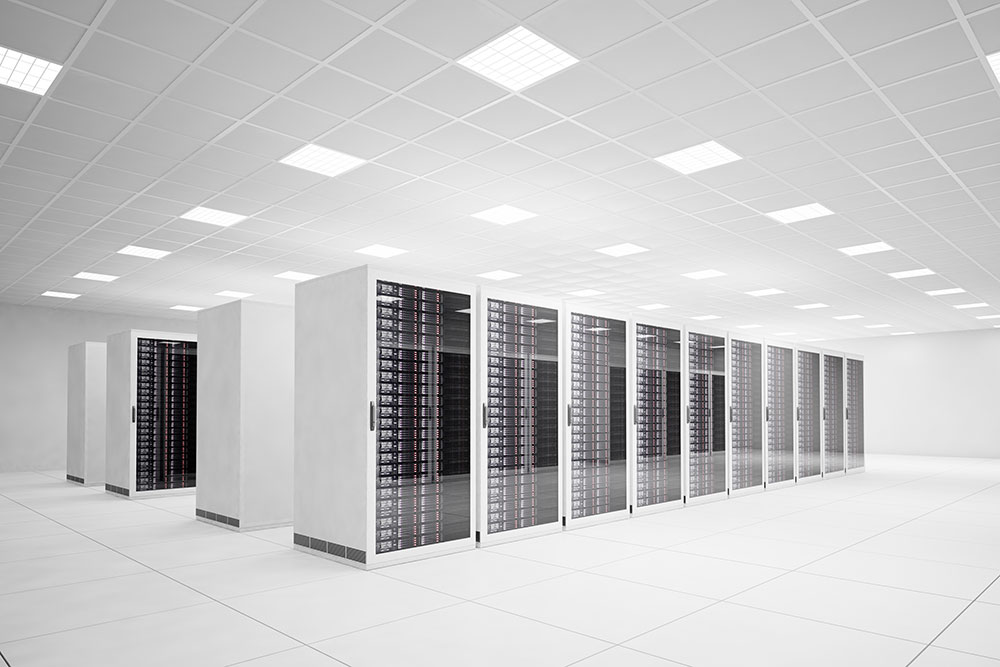
Google’s Road to Reducing Data Centre Energy Consumption
As many of you would know, data centres are the physical homes to servers used for web hosting (and the digital homes to your websites). Data centres also require more than an insane buttload of energy; in fact, they’re notorious for their energy consumption. In 2013, Google had around 13 data centres globally and they used 260 million watts of power, which accounts to 0.01% of global energy (according to Storage Servers).
However, Google is, as of recently, one step closer to fixing the problem. By applying machine learning to Google data centres, they saw a huge 40% cut in energy usage. The AI (Artificial Intelligence) system was built by British AI company, DeepMind, to control certain parts of its data centres to make its vast server farms more environmentally friendly. It was bought by Google for more than US$600 million (AUD$798 million) about two years ago, and is famous for developing AI technology advanced enough to beat the world best (human) player of the board game Go (no, not Pokemon Go).
“By applying machine learning to Google data centres, they were saw a huge 40% cut in energy usage.”
The Problem
One of the factors of energy consumptions in a data centre is cooling. Just as many of your mobile phone or laptop generate heat, so do data centres — except, imagine over a million laptops overheating in a gigantic warehouse. For servers to perform at an optimal level as well as reduce the amount of operating problems, these heating pieces of machinery need to be cooled. This is usually done via large industrial equipment such as pumps, chillers, and cooling towers, but these technologies are difficult to operate optimally for several reasons
The Solution
In an effort to address the cooling problem, Google teamed up with DeepMind to apply machine learning to their data centres around two years ago. Together, they created a more efficient and adaptive framework to understand data centre dynamics and optimise efficiency by “using a system of neural networks trained for different operating scenarios and parameters”.
Basically, they used historical data collected by thousands of sensors within the test data centre (such as temperatures, power, pumps speeds, setpoints, etc.), to train an ensemble of deep neural networks on the average future Power Usage Effectiveness (PUE).
They then trained an additional two ensembles to predict the future temperatures of the data centre over the next hour, to ensure that they don’t go beyond any operating constraints.
They tested the model by deploying it on a live data centre. The graph below shows the PUE for the day, as well as when they used machine learning (ML):
Google and DeepMind plan to apply this to other challenges in the data centre environment in the coming months. As well as this, they have indicated other possible future applications including:
- Improving power plant conversion efficiency
- Reducing semiconductor manufacturing energy and water usage
- Helping manufacturing facilities increase their rate of production
“A phenomenal step forward for any large scale energy-consuming facility.”